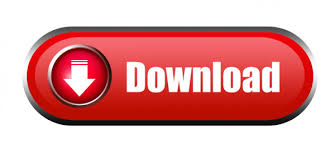
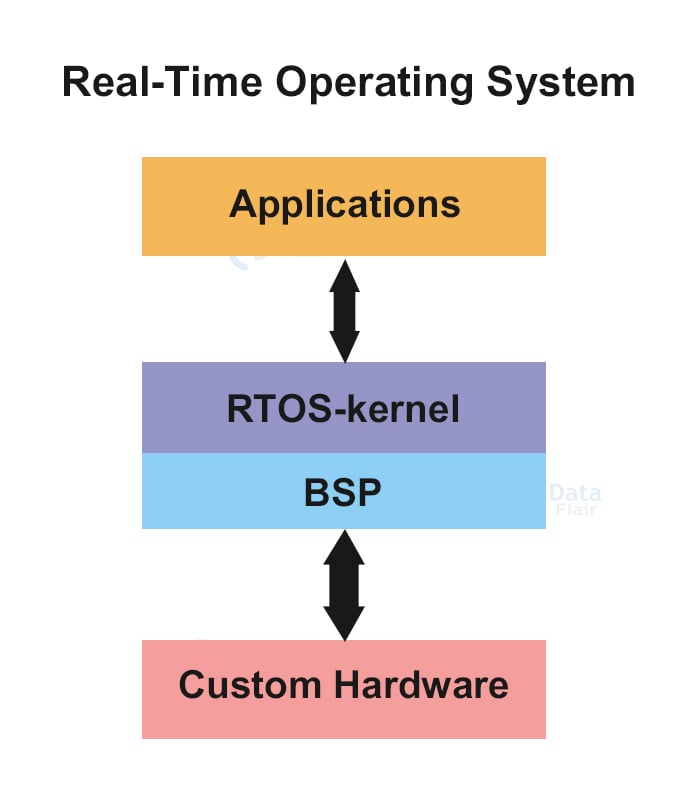
The reducer can produce output files which can serve as input into another MapReduce job, thus enabling multiple MapReduce jobs to chain into a more complex data processing pipeline. All the grouped values entering the reducers are sorted by the framework. The MapReduce framework collects all the key-value pairs produced by the mappers, arranges them into groups with the same key and applies the reduce function. # Split the text into words and yield word,1 as a pair # Map function, is applied on a partition
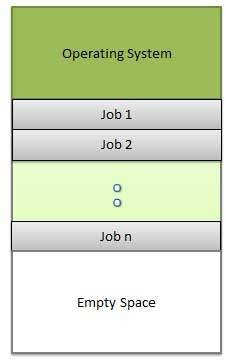
Below, we see an example of a simple mapper that takes the input partition and outputs each word as a key with value 1.
BATCH PROCESSING OPERATING SYSTEM EXAMPLE CODE
The user only needs to define the code inside the mapper. The mapper can generate any number of key-value pairs from a single input (including zero, see the figure above). The map task (mapper) is called once for every input partition and its job is to extract key-value pairs from the input partition. This is hidden from the user, but it is important to be aware of it because the number of partitions can affect the speed of execution. In order to increase parallelization, each directory is made up of smaller units called partitions and each partition can be processed separately by a map task (the process that executes the map function). In Hadoop, the typical input into a MapReduce job is a directory in HDFS. It also has its own distributed file storage called HDFS. Hadoop, along with its many other features, had the first open-source implementation of MapReduce. Everything except the interface of the functions is programmable by the user. The map phase generates key-value data pairs from the input data (partitions), which are then grouped by key and used in the reduce phase by the reduce task. When writing a MapReduce job we have to follow the strict interface (return and input data structure) of the map and the reduce functions. The computation performance of MapReduce comes at the cost of its expressivity. Each node runs (executes) the given functions on the data it has in order the minimize network traffic (shuffling data). To run a MapReduce job, the user has to implement two functions, map and reduce, and those implemented functions are distributed to nodes that contain the data by the MapReduce framework. This is ideal because the code is much smaller than the data. This way, the data stays on the same node, but the code is moved via the network. In order to decrease the duration of our distributed computation, MapReduce tries to reduce shuffling (moving) the data from one node to another by distributing the computation so that it is done on the same node where the data is stored. This is different from vertical scaling, which implies increasing the performance of a single machine. This allows the computation to handle larger amounts of data by adding more machines – horizontal scaling. Today, it is implemented in various data processing and storing systems ( Hadoop, Spark, MongoDB, …) and it is a foundational building block of most big data batch processing systems.įor MapReduce to be able to do computation on large amounts of data, it has to be a distributed model that executes its code on multiple nodes. MapReduce is a programming model that was introduced in a white paper by Google in 2004. Doing this as a daily job could give insights into customer trends. Usually, the job will read the batch data from a database and store the result in the same or different database.Īn example of a batch processing job could be reading all the sale logs from an online shop for a single day and aggregating it into statistics for that day (number of users per country, the average spent amount, etc.). It runs the processing code on a set of inputs, called a batch. Batch processing is an automated job that does some computation, usually done as a periodical job. There are a lot of use cases for a system described in the introduction, but the focus of this post will be on data processing – more specifically, batch processing. MapReduce is a framework that allows the user to write code that is executed on multiple nodes without having to worry about fault tolerance, reliability, synchronization or availability. Writing distributed systems is an endless array of problems, so people developed multiple frameworks to make our lives easier.
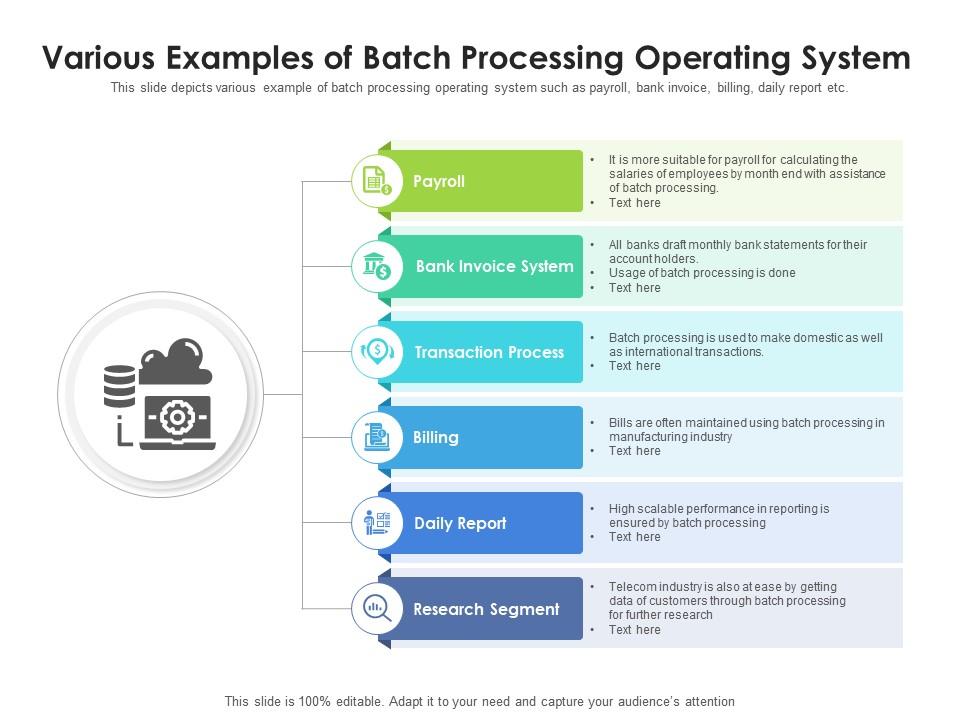
Therefore, there was a need to develop code that runs on multiple nodes. Today, the volume of data is often too big for a single server – node – to process.
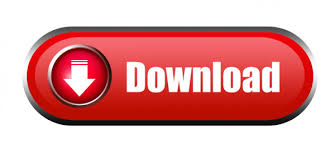